Use AI To Make Customer Feedback Analytics More Actionable
AI transforms customer feedback into actionable insights, optimizing product development and enhancing user satisfaction through data analysis.
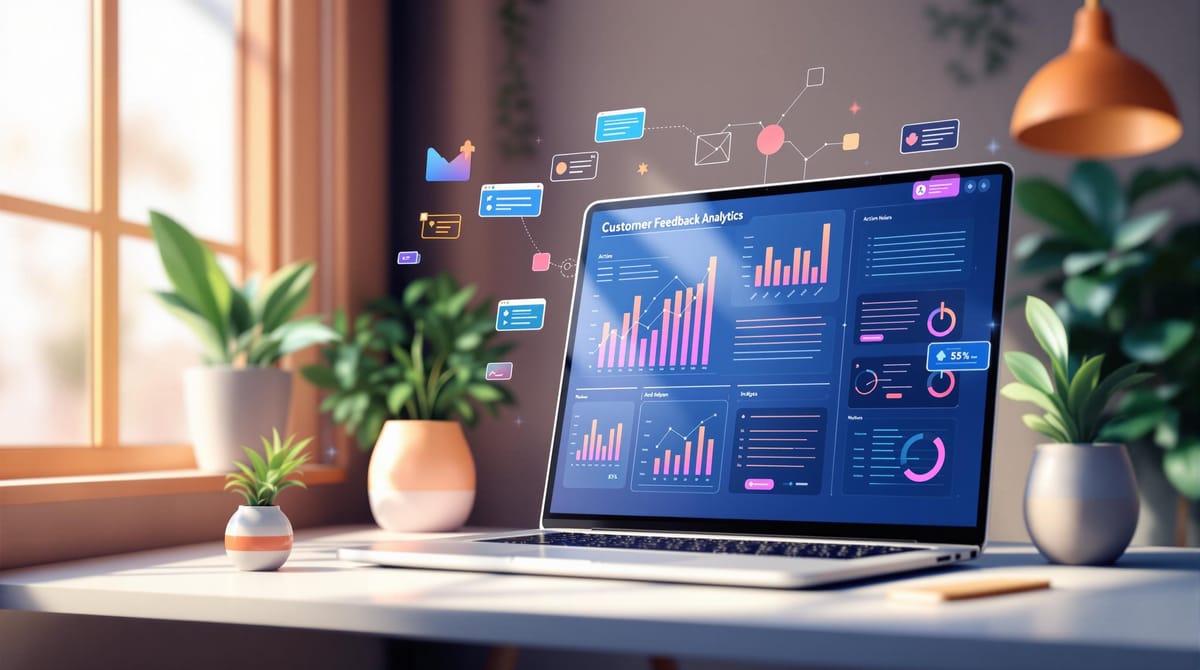
AI can transform overwhelming customer feedback into clear, actionable insights - faster and more efficiently than manual methods.
Here’s how AI makes this possible:
- Organizes Feedback: Natural Language Processing (NLP) groups similar issues, like feature requests or bug reports.
- Analyzes Emotions: Sentiment analysis identifies customer satisfaction levels and flags urgent problems.
- Predicts Trends: AI anticipates future customer needs, helping prioritize product updates.
- Ranks Impact: Feedback is sorted by business impact, ensuring teams focus on what matters most.
How We Turned Customer Feedback into Actionable Insights ...
How AI Sorts and Ranks Customer Feedback
AI turns scattered customer feedback into clear, actionable insights, helping product teams quickly pinpoint and address key customer needs. Let’s break down how AI categorizes topics, analyzes emotions, and prioritizes issues based on their impact.
Grouping Feedback by Topic with NLP
Natural Language Processing (NLP) helps automatically sort feedback by identifying key words and phrases. It spots common themes, feature requests, and issues by analyzing patterns in language, context, and customer intent. This way, similar feedback gets grouped together.
Here’s how AI organizes feedback into useful categories:
Category Type | What AI Analyzes | Business Value |
---|---|---|
Feature Requests | Mentions of product functionality | Helps guide product roadmaps |
Bug Reports | Descriptions of technical issues | Speeds up issue resolution |
User Experience | Comments on interface and workflow | Improves usability |
Performance Issues | Feedback on speed and reliability | Highlights system bottlenecks |
Measuring Customer Emotions in Feedback
AI doesn’t just group feedback - it also evaluates the tone behind customer comments. Sentiment analysis digs deeper than simple positive or negative labels, identifying the intensity of emotions like frustration, excitement, or confusion. By analyzing tone and context, AI provides insights into customer satisfaction levels, helping teams prioritize responses based on urgency.
Ranking Feedback by Business Impact
After grouping and analyzing sentiment, AI ranks feedback by its business impact. It measures both the frequency and severity of issues. For instance, if many customers report the same problem, AI connects these recurring concerns to planned product updates. By pulling in real-time data - like reactions from product demos or support interactions - AI highlights the issues that most affect business outcomes. This ensures product teams focus on changes that deliver the greatest results.
Finding Key Patterns in Customer Feedback
AI is incredibly effective at identifying patterns in large volumes of customer feedback - patterns that might go unnoticed by humans. By analyzing both historical data and real-time inputs, AI helps product teams detect trends, link feedback to user behavior, and even predict future needs.
Spotting New Trends and Problems
AI continuously scans feedback across various channels, using natural language processing (NLP) to identify emerging issues or opportunities. This automated process allows teams to detect problems early and recognize trends that could be amplified for growth.
Take Vodafone New Zealand, for example. They used AI-powered text analytics to process customer feedback more efficiently. This system pinpointed recurring issues and highlighted new trends, cutting down on manual work and speeding up decision-making based on data. These insights help tie feedback directly to user behavior.
Here’s a breakdown of how AI categorizes feedback patterns by their impact:
Pattern Type | AI Detection Method | Business Impact |
---|---|---|
Emerging Issues | Monitoring spikes in negative sentiment | Preventing problems early |
Feature Requests | Grouping similar suggestions into clusters | Shaping product roadmaps |
Usage Patterns | Analyzing user interaction behaviors | Improving user experience |
Success Stories | Tracking positive sentiment across channels | Highlighting growth opportunities |
Linking Feedback to User Actions
AI doesn’t just collect feedback - it connects it to specific user behaviors and product interactions. This reveals the “why” behind customer comments.
For instance, Atom Bank used AI to analyze complaints alongside user behavior. By linking negative feedback to specific mortgage-related actions, they reduced support calls by 69%.
Using Past Feedback to Plan Ahead
AI’s predictive analytics build on past trends to help product teams forecast customer needs and identify upcoming trends. These predictions improve service responsiveness and personalization.
Spotify is a great example of this. By analyzing listening habits and feedback, their AI creates personalized playlists like Discover Weekly. Processing millions of data points, the system predicts which songs will resonate with users, boosting engagement and satisfaction.
Converting Feedback into Product Changes
After gathering insights with AI, product teams can shift to action. AI simplifies the process of turning feedback into updates, helping teams deliver improvements more efficiently. Here's how AI-driven insights directly lead to product updates.
Getting AI Product Suggestions
AI pinpoints specific areas for improvement. For example, Mention reviewed hundreds of G2 and Capterra reviews, combined with NPS scores and feedback from churned customers, to create a concise 10-minute report. This report highlighted key areas for improvement, shaping their development plans for Q3 and Q4, with a focus on software usability.
Fixing Common Customer Problems Faster
AI speeds up problem-solving by identifying recurring issues. Kenko Tea, for instance, used AI sentiment analysis to uncover frequent complaints about their loose-leaf matcha packaging. They redesigned the packaging, which led to a 50% drop in negative reviews and a 10% increase in customer satisfaction.
Making Products Match User Needs
AI tailors products to better align with user preferences.
"AI can help us forecast trends and opportunities by analyzing the key topics and keywords that appear most often in customer feedback"
explains Sam Speller, Founder and CEO of Kenko Tea. One company, noticing growing interest in matcha-based recipes, expanded its recipe offerings. They added new website content and email campaigns centered on these recipes, strengthening customer loyalty.
"By analyzing historical feedback data, AI models can predict which features or aspects of our tools are likely to cause user frustration or delight"
says Alari Aho, CEO and founder of Toggl. This allows teams to address potential issues before they escalate, ensuring a smoother user experience.
Tips for Using AI with Customer Feedback
Choosing the Right AI Tools
Select AI tools that align with your feedback goals. Marc Dufraisse advises: "First, clearly determine what you want to achieve with the NLP tool. This could be understanding overall customer sentiment, extracting specific themes or issues, or measuring changes in customer satisfaction over time. Your goals will guide you in selecting a tool that is best suited to your specific needs."
When assessing AI solutions, consider these three factors:
- Integration Options: Tools like BuildBetter connect to over 100 data sources, offering thorough feedback analysis.
- Scalability: Check if the tool's pricing and capacity can handle increasing data volumes.
- Feature Fit: Ensure the tool provides the features you need, such as advanced sentiment analysis for product reviews.
Improving AI Accuracy
Accurate results depend on well-prepared data. Companies like ICUC.Social improve their feedback analysis by consistently formatting, normalizing, and retraining models with verified data.
"Once our human analysts review these AI reports, they interpret the insights to double-check accuracy and identify more nuanced responses. This is key to connecting the dots between raw data and actionable advice because a robot simply won't have the contextual knowledge needed to interpret certain trends properly."
These steps help balance automated insights with human expertise for better results.
Balancing AI and Human Analysis
Decide when to rely on AI versus human input based on the type of feedback.
"AI isn't yet capable of context and nuance. Our human reps are still vital for understanding the 'why' behind the sentiment and for adding the personal touch."
Here’s a quick comparison:
Analysis Type | Best for AI | Best for Humans |
---|---|---|
High-Volume Tasks | Processing large datasets | Extracting deeper insights |
Pattern Detection | Spotting recurring themes | Grasping cultural or contextual nuances |
Response Time | Quick categorization | Detailed interpretation |
Accuracy Check | Initial screening | Final validation |
Combining AI with human input ensures more precise and actionable feedback analysis.
Conclusion: Better Products Through AI Feedback Analysis
Key Takeaways
AI-driven feedback analysis is changing how companies understand and act on customer insights. While only 28% of customer service professionals currently use AI for this purpose, those who do report notable improvements.
Here’s why AI feedback analysis stands out:
- Speed and Efficiency: AI processes unstructured data in record time compared to manual efforts.
- Unbiased Pattern Recognition: AI identifies patterns without the influence of human bias, making it easier to implement data-backed product updates.
- Practical Insights: Real-world examples show that AI insights directly lead to product refinements and measurable outcomes.
As technology evolves, AI feedback analysis will continue to improve and provide even more actionable insights.
The Future of AI Feedback Analysis
Looking ahead, several advancements will shape the next generation of AI-driven feedback tools:
- Deeper Integration: AI will work alongside IoT and AR technologies, offering a more holistic view of customer feedback.
- Smarter Language Processing: Enhanced NLP models will better interpret subtle context and nuances in customer input.
"AI's limitations include potential misinterpretation of nuanced feedback and a lack of creativity and emotional understanding... a robot simply won't have the contextual knowledge needed to interpret certain trends properly." – Sally Bannerman, Director of Product Marketing at ICUC.Social
To stay ahead, businesses should focus on these essential steps:
- Strengthen data privacy measures to comply with regulations like GDPR and CCPA.
- Regularly audit and update AI models to ensure accuracy and relevance.
- Train employees to improve their understanding and use of AI tools.
- Seamlessly integrate AI systems with existing workflows.